|
A Powerful Tool for Data Mining Support Vector Machines (SVMs) are a set of supervised learning methods used for classification and regression analysis. They are particularly effective in high-dimensional spaces and are widely used in various fields, including bioinformatics, image recognition, and natural language processing. How SVMs Work Hyperplane: SVMs find a hyperplane in a high-dimensional space that separates data points into two classes. Margin: The goal is to maximize the margin between the hyperplane and the nearest data points (support vectors). Kernel Trick: SVMs can map data into a higher-dimensional space using a kernel function, allowing them to handle non-linearly separable data.
Key Advantages of SVMs High Accuracy: SVMs often achieve high accuracy, especially in high-dimensional spaces. Robustness: They are relatively insensitive to outliers and noise. Versatility: SVMs can handle both linear and non-linear classification problems. Efficiency: They can be efficient Telegram Number for large datasets, especially with sparse feature vectors. Applications of SVMs Image Classification: SVMs have been successfully used for tasks such as object detection and facial recognition. Text Classification: They can classify text documents into categories like spam or non-spam. Bioinformatics: SVMs are used for protein structure prediction and gene expression analysis. Financial Forecasting: They can predict stock prices and market trends. Types of SVMs Linear SVM: For linearly separable data. Nonlinear SVM: Uses kernel functions to handle non-linearly separable data.
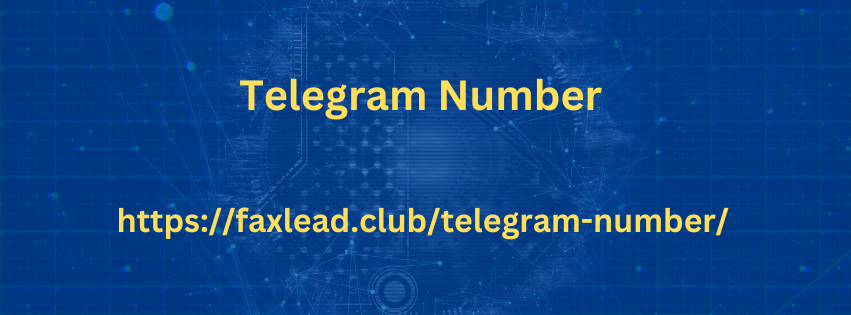
C-SVM: Controls the trade-off between maximizing the margin and minimizing the number of misclassifications. ν-SVM: Controls the number of support vectors. Challenges and Considerations Computational Complexity: SVMs can be computationally expensive for large datasets, especially with complex kernel functions. Hyperparameter Tuning: Selecting the appropriate kernel function and hyperparameters can be challenging. Interpretability: The decision boundaries of SVMs can be difficult to interpret, making it harder to understand how the model makes predictions. In conclusion, SVMs are a powerful tool for data mining, offering high accuracy and versatility. However, their computational complexity and the need for careful hyperparameter tuning should be considered when applying them to specific problems.
|
|